Critical Systems Engineering - Socio-technical Vehicle Systems (The Car that Cares)
Due to the increasing automation human-machine-interaction becomes more and more important. In this project, the socio-technical relationship between the individual driver and the technical systems of the vehicle is investigated regarding a future car “that cares”. Areas of research also include the surrounding traffic and road infrastructures.
The scope of the CSE subproject The Car That Cares (CtC) is the development of socio - technical vehicle systems, which continuously adapt to internal (e.g. driver 's health, current tasks) and external conditions (e.g. weather, traffic) to offer a situation-related cooperative interaction with the driver of the car. The project addresses elderly drivers (aged 65 and over) who are increasing in numbers due to the demographic change. On the one hand, elderly drivers have a higher risk of accidents due to various restrictions accompanying the decline in age (such as perception or attention shift) that are crucial for driving. On the other hand, elderly drivers have a personal desire and a social need to stay mobile and independent as long as possible. High-resolution information about the drivers ‘cognitive status is essential to prevent overload situations and to enhance their performance.
Aim
We want to assess the cognitive, emotional, and health status of the driver within the car by a multi-sensor approach. Systems are developed to support the driver and provide recommendations to improve safety, comfort, and health through adaptive multimodal user interfaces.
The focus of our research group is the development of robust procedures for the detection and context-specific modulation of the neurocognitive status of the elderly driver. We want to improve processing capabilities and reaction times to support appropriate and safe actions even in traffic situations with increasing complexity and under time pressure.
Approach
Therefore, our research group develops and refines systems for the detection and enhancement of cognitive performance and evaluates their suitability for application:
- We develop a special mobile fNIRS system that makes it possible to measure cognitive load reliably in realistic environments. The mobile fNIRS will be more robust against environmental influences (movement of the subject, stray light) and achieve higher signal quality.
- Transcranial alternating current (tACS) is investigated as a method for modulating the neurocognitive driver's status, also for the elderly. In tACS, electrodes glued to the head generate an alternating current field that influences intrinsic brain waves. tACS can modify certain brain waves and thus, among other things, increase cognitive capabilities (e.g. in learning tasks). We investigate the applicability of tACS to the driving context. Current scenarios are the enhancement of attention or reaction times during monotonous or tedious driving tasks.
- In the Living Lab State Characterization and Identification (LL SCI) neurocognitive measurement methods for studies in the driving context are prepared. These methods are going to be used to evaluate previous results and to gain further insights into the neuronal activities in complex driving situations. In addition to systems like fNIRS and tACS, imaging techniques such as MEG and MRI are used and appropriate MRI and MEG suitable driving simulator hardware (steering wheels and pedals) are developed. These components will be integrated into a processing system together with a driving simulator and used in studies.
Grants and cooperations
The interdisciplinary research center for the design of safety-critical socio-technical systems investigated the role of humans in the control of complex transport systems on land and water. Cooperation partners are OFFIS e.V. in Oldenburg, DLR Institute for Traffic Systems Engineering in Braunschweig and the network SafeTRANS. Afterwards, the funding has been extended for a second phase by the state of Lower Saxony with EUR 2 million.
Publications
2018 | |
[6] | N. Volkening, A. Unni, S. Becker, JW. Rieger, S. Fudickar, A. Hein. Development of a Mobile Functional Near-infrared Spectroscopy Prototype and its Initial Evaluation: Lessons Learned. In: Proceedings of the 11th PErvasive Technologies Related to Assistive Environments Conference on - PETRA '18. ACM Press; 2018:214–221. |
[5] | N. Volkening, A. Unni, JW.. Rieger, S. Fudickar, A. Hein. Development of a Mobile Functional Near-Infrared Spectroscopy Prototype. In: AM.J.. Skulimowski, Z. Sheng, S. Khemiri-Kallel, C. Cérin, CH. Hsu, eds. Internet of Vehicles. Technologies and Services Towards Smart City. Cham: Springer International Publishing; 2018:146–161. |
[4] | B. Löffler, HI. Stecher, S. Fudickar, D. de Sordi, F. Otto-Sobotka, A. Hein, C. Herrmann. Counteracting the Slowdown of Reaction Times in a Vigilance Experiment with 40-Hz Transcranial Alternating Current Stimulation. IEEE - Transactions on Neural Systems & Rehabilitation Engineering. 2018. |
2017 | |
[3] | S. Blum, S. Debener, R. Emkes, N. Volkening, S. Fudickar, MG. Bleichner. EEG Recording and Online Signal Processing on Android: A Multiapp Framework for Brain-Computer Interfaces on Smartphone. BioMed Research International Hindawi. 2017;2017:12. |
2016 | |
[2] | N. Volkening, A. Unni, BS. Löffler, S. Fudickar, JW.. Rieger, A. Hein. Characterizing the Influence of Muscle Activity in fNIRS Brain Activation Measurements. In: IFAC-PapersOnLine. 2016;49(11):84–88. |
2015 | |
[1] | BS. Löffler, S. Fudickar, CS.. Herrmann, A. Hein. Ein Blick in das Gehirn: Messung und Verbesserung der Fahrleistung älterer Autofahrender mit Hilfe von Neuroimaging und Elektrostimulation. In: TZKG. Carolin Wienrich, ed. Trends in Neuroergonomics. Berlin: Universitätsverlag der TU Berlin; 2015:166–169. |
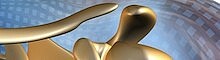
- Research
- AI und Deep Learning in Medicine
- Medical Image Processing and VR-Simulation
- Integration and Utilisation of Medical Data
- Sensor Data Analysis for Assistive Health Technologies
- Medical Image Computing and Artificial Intelligence
- Medical Data Science Lab
- Medical Deep Learning Lab
- Medical Data Engineering Lab
- Junior Research Group Diagnostics and Research of Movement Disorders
Contact


