MDMLA: Multi-task Deep Learning for Large-scale Multimodal Biomedical Image Analysis (BMBF)
It is a common scientific approach to investigate physical objects using different, complementary experimental measurements, which differ in their spatial and temporal resolution, dimensionality and modality specificity and lead to a large amount of image data to be evaluated.
Within this interdisciplinary project, a generalistic deep learning framework will be developed and implemented, which enables efficient processing and analysis of multimodal and longitudinal biomedical data across modalities and domains. In close cooperation with experts from the user and machine learning domain (DESY, HZG, Syntellix, IMT, IMI) new multitask deep learning methods will be developed, which holistically combine the different complementary tasks and thus transfer knowledge across the individual analyses. Subsequently, generic structures within the developed network architectures will be identified in order to design a unified, data-driven framework that can be applied to a variety of different domains, modalities and tasks.
The developed methods and tools are applied to a variety of longitudinal and multimodal data sets from preclinical studies and clinical applications, e.g. for the investigation of bone implants. The implementation of the methods on a suitable high performance computing platform will lead to a long-term service that will be available to a large number of scientific users.
Within the scope of this project, new approaches for deep learning based image registration and multi-task learning will be developed at IMI. In particular, we plan to decouple feature extraction and geometric modelling to enable the best possible solutions for a variety of tasks (reconstruction, registration, segmentation) and modalities (laboratory computed tomography (labCT), synchrotron radiation micro-CT (SRμCT), magnetic resonance imaging (MRI), small angle X-ray scattering (SAXS) and histology).
BMBF Project Funding (2020-2023) 1‘170‘157€ (507‘163€ UzL)
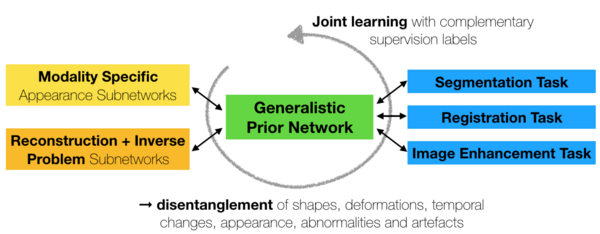
Selected Publications
- Heinrich M.P., Hansen L.
Unsupervised learning of multimodal image registration using domain adaptation with projected Earth Move's discrepancies
Medical Imaging with Deep Learning (MIDL 2020, Montreal, short-paper)
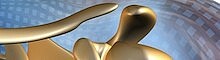
- Research
- AI und Deep Learning in Medicine
- Medical Image Processing and VR-Simulation
- Integration and Utilisation of Medical Data
- Sensor Data Analysis for Assistive Health Technologies
- Medical Image Computing and Artificial Intelligence
- Medical Data Science Lab
- Medical Deep Learning Lab
- Medical Data Engineering Lab
- Junior Research Group Diagnostics and Research of Movement Disorders