AutoSAFE: AI-guided assistance system for the diagnosis of infantile forearm fractures. (BMBF)
AutoSAFE is a project to improve the diagnosis of pediatric forearm fractures using AI-assisted image acquisition and analysis of fracture sonography.
Wrist related forearm fractures or forearm contusions are common causes of medical presentation in childhood and adolescence. As part of standard diagnostics, a double X-ray examination is performed in such cases, which is accompanied by exposure to ionizing radiation. In view of the increased radiation sensitivity of infantile tissue, a reduction of X-ray diagnostics while maintaining diagnostic quality is desirable.
Fracture sonography (ultrasound examination of the bone) can be used to assess the fracture and thus avoid up to 80% of radiographs. In addition, sonography offers a rapid, safe, "point-of-care" diagnostic procedure that is free of side effects and is often available to office-based physicians. A standardized approach to diagnosis is the Wrist-SAFE (Sonographic Algorithm for Fracture Evaluation) algorithm (Ackermann et al. 2019), which is based on the structured acquisition and analysis of 6 standard planes of the radius and ulna.
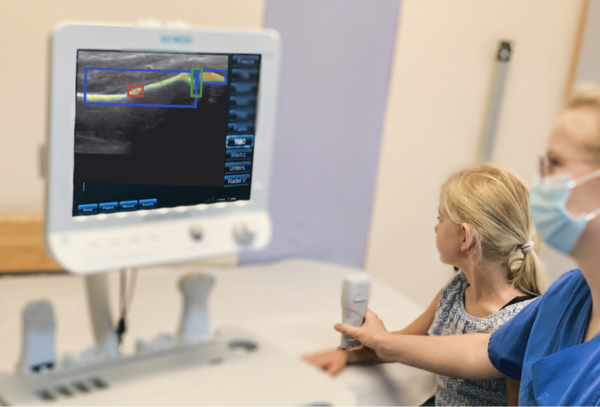
The AutoSAFE project aims to partially automate the Wrist-SAFE algorithm to support medical professionals in the diagnosis of forearm fractures near the wrist.
To support medical professionals, an assistance system based on AI (artificial intelligence) will be developed to facilitate the diagnosis:
- Based on temporal ultrasound images, a navigation algorithm will be developed to guide examining physicians in real time and offer recommendations for optimal acquisition of standard planes.
- The image of the standard planes is automatically supplemented with segmentations of relevant structures as well as the possible fracture, thus enabling a well-founded diagnosis.
In addition to the University of Lübeck, UKSH Lübeck and the sonography startup ThinkSono are involved in the project.
BMBF project funding (2021-2024) 790.567€ (UzL 222.000€)
Selected Publications
1.Kainz, B., Heinrich, M.P., Makropoulos, A. et al.: Non-invasive diagnosis of deep vein thrombosis from ultrasound imaging with machine learning. npj Digit. Med. 4, 137 (2021). https://doi.org/10.1038/s41746-021-00503-7
2. Tanno R., Makropoulos A., Arslan S., Oktay O., Mischkewitz S., Al-Noor F., ... & Heinrich M.P.: AutoDVT: Joint real-time classification for vein compressibility analysis in deep vein thrombosis ultrasound diagnostics.In: International Conference on Medical Image Computing and Computer-Assisted Intervention 2018 (pp. 905-912). Springer, Cham.
3. Kahl, F., Tüshaus, L, Kaiser, M.-M., Wessel, L.: Nachweis kindlicher distaler Unterarmfrakturen mittels Knochensonographie - ein strahlenarmes Diagnostikkonzept. 100. Jahrestagung der Deutschen Gesellschaft für Kinderheilkunde und Jugendmedizin, Berlin, 09.09. – 12.09.2004. Vortrag als Abstract publiziert in: Monatsschrift Kinderheilkunde 2004, 152, Suppl. 1, 263.
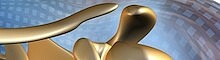
- Research
- AI und Deep Learning in Medicine
- Medical Image Processing and VR-Simulation
- Integration and Utilisation of Medical Data
- Sensor Data Analysis for Assistive Health Technologies
- Medical Image Computing and Artificial Intelligence
- Medical Data Science Lab
- Medical Deep Learning Lab
- Junior Research Group Diagnostics and Research of Movement Disorders
- Former Medical Data Engineering Lab