Automatic Patient Individual Contrast Agent Dose Optimization (iQ-CM)
Contrast agents are used in medical imaging to visualize anatomical structures (fig. 1) and to make physiological parameters e.g. perfusion measurable. They usually consist of substances whose toxicity can be mitigated biochemically, still side effects occur in numerous cases, which sometimes are severe. Most radiologists use universal doses of contrast agents for contrast enhanced imaging, that may exceed the requirements in some cases. The aim of this project is to develop methods and algorithms to optimize the patient individual contrast agent doses for contrast-enhanced computed tomography (CT) examinations, which can reduce the average dose required.
The focus of our work lies in the development and evaluation of multi-parametric models on the basis of patient individual parameters such as age, gender, blood pressure, pulse rate, as well as examination-specific parameters such as injection speed of the bolus. Model-based and machine learning methods will be used to analyze and describe complex, multi-parametric correlations in order to perform a patient-specific optimized prediction of contrast agent requirements.
The predictive power of the model-based methods and the machine learning methods will be evaluated on the basis of clinical study that will be carried out at the Clinic for Radiology and Nuclear Medicine in the context of this project.
The project is realized in collaboration with the Clinic for Radiology and Nuclear Medicine of the University Medical Center Schleswig-Holstein, Campus Lübeck, and the company IMAGE Information Systems Europe GmbH and is funded by the German Federal Ministry of Education and Research (BMBF).
Project Team
M.Sc. Kira Leane Soika
Prof. Dr. rer. nat. Heinz Handels
Cooperation Partners
Prof. Dr. med. J. Barkhausen, PD Dr. med. P. Hunold und M. Schürmann
Klinik für Radiologie und Nuklearmedizin
Universitätsklinikum Schleswig-Holstein, Campus Lübeck
Dr. med. A. Bischof, Dr. rer. nat. C. Godemann, H. Marien, E. Virtel und S. Schülke
IMAGE Information Systems Europe GmbH
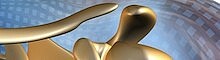
- Research
- AI und Deep Learning in Medicine
- Medical Image Processing and VR-Simulation
- Integration and Utilisation of Medical Data
- Sensor Data Analysis for Assistive Health Technologies
- Medical Image Computing and Artificial Intelligence
- Medical Data Science Lab
- Medical Deep Learning Lab
- Medical Data Engineering Lab
- Junior Research Group Diagnostics and Research of Movement Disorders