Deep learning methods for image analysis have shown to excel at many tasks such as segmentation, detection and classification. However, they usually require a large Deep Learning-based Generative Models for Unsupervised Anomaly Detection in Medical Images
Deep learning methods for image analysis have shown to excel at many tasks such as segmentation, detection and classification. However, they usually require a large amount of annotated images to learn a particular task. For example, if the aim is to segment brain tumors in MRI images, a deep learning algorithm would require hundreds or thousands of brain MRIs with expert annotations of the tumor tissue. Such datasets are rarely accessible in the medical field since the annotation of images is a complicated and time consuming process.
In this project, the focus lies on detecting pathologies and anomalies in medical images without using ground truth segmentations for training, so called unsupervised learning. The main idea is to learn and model the variability of healthy tissue appearance of a certain data domain, so that pathologies are recognized as deviations from the learned norm. Deep learning-based generative models such as variational autoencoders or GANs are a tool of choice in this project, since they enable the learning of complex representations and distributions.
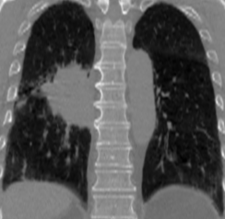
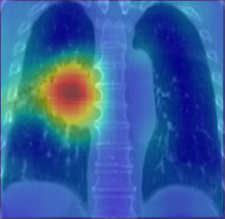
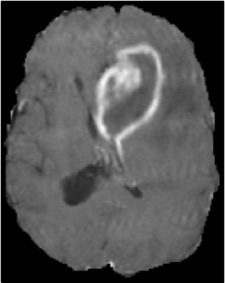
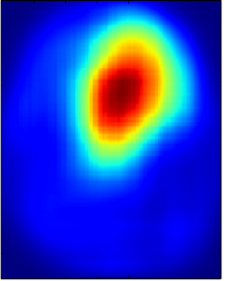
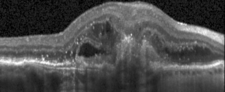
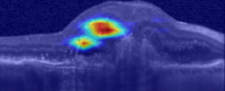
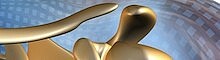
- Research
- AI und Deep Learning in Medicine
- Medical Image Processing and VR-Simulation
- Integration and Utilisation of Medical Data
- Sensor Data Analysis for Assistive Health Technologies
- Medical Image Computing and Artificial Intelligence
- Medical Data Science Lab
- Medical Deep Learning Lab
- Medical Data Engineering Lab
- Junior Research Group Diagnostics and Research of Movement Disorders