Integrated 4D Segmentation and Registration of Spatio-temporal Image Data
The introduction of spatio-temporal tomographic image data enabled the analysis of dynamic physiological processes like heart beat or respiratory lung motion. However, its potential is not yet fully capitalized on. A comprehensive diagnostic and therapeutic usage of 4D data requires on the one hand a delineation of clinically relevant structures (segmentation), on the other hand an explicit description of motion characteristics (registration). Classical approaches regard both problems independently, however, a mutual dependency between them exists.
Aim of this project is the development of simultaneous segmentation and registration approaches that allow for a modeling of the mutual dependency. A-priory knowledge about physiology and motion dynamic is introduced by formulating appropriate side conditions. Methods for an automatic parameter detection and refinement allow for an adaption of the proposed segmentation and registration algorithm to specific medical applications. Moreover, the introduction of interaction tools enables the user-driven correction and improvement of results.
The base idea of the approach is illustrated in the figure below. Looking at two 3D images of a spatio-temporal data set (called reference and target image), a given segmentation of the reference image is assumed. Aim is on the one hand the calculation of a segmentation of the target image, on the other hand the motion estimation between target and reference image. An additional term ensures the consistency of segmentation and registration by comparing target and transformed reference segmentation.
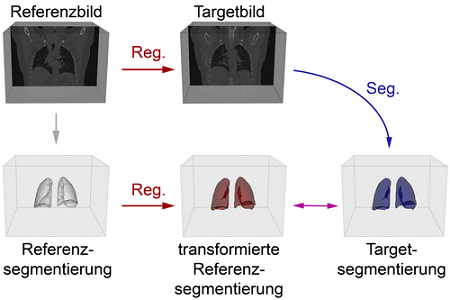
The approaches developed in this project are evaluated for the simultaneous segmentation and motion estimation of lung and liver on the base of clinical CT data.
The project is funded by Deutsche Forschungsgemeinschaft (DFG: EH 224/3-1).
Selected Publications
- A. Schmidt-Richberg, H. Handels, J. Ehrhardt
Integrated Segmentation and Non-linear Registration for Organ Segmentation and Motion Field Estimation in 4D CT Data.
Methods Inf Med, 48(4): 334–339, Jan 2009. - A. Schmidt-Richberg, J. Ehrhardt, R. Werner, H. Handels
Direction-Dependent Regularization for Improved Estimation of Liver and Lung Motion in 4D Image Data.
In: SPIE Medical Imaging 2010, San Diego, USA, Vol. 7623, 76232Y, 2010. - A. Schmidt-Richberg, J. Ehrhardt, R. Werner, H. Handels
Slipping Objects in Image Registration: Improved Motion Field Estimation with Direction-dependent Regularization.
In: G.-Z. Yang et al. (eds.): Medical Image Computing and Computer-Assisted Intervention - MICCAI 2009, London, LNCS Vol. 5761, 755–762, 2009. - J. Ehrhardt, A. Schmidt-Richberg, H. Handels
Simultaneous Segmentation and Motion Estimation in 4D-CT Data Using a Variational Approach.
In: J.M. Reinhardt et al. (eds.): Image Processing, SPIE Medical Imaging 2008, San Diego, Vol. 6914, 37-1–37-10, 2008. - J. Ehrhardt, A. Schmidt-Richberg, H. Handels
A Variational Approach for Combined Segmentation and Estimation of Respiratory Motion in Temporal Image Sequences.
IEEE International Conference on Computer Vision 2007, ICCV 2007, Rio de Janeiro, Brazil, CD-ROM-Proceedings, IEEE Catalog Number CFP07198-CDR (ISBN 978-1-4244-1631-8), 2007.
Project Team
Dipl.-Inf. Alexander Schmidt-Richberg
Dr. Jan Ehrhardt
Prof. Dr. Heinz Handels
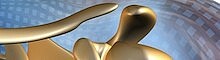
- Research
- AI und Deep Learning in Medicine
- Medical Image Processing and VR-Simulation
- Integration and Utilisation of Medical Data
- Sensor Data Analysis for Assistive Health Technologies
- Medical Image Computing and Artificial Intelligence
- Medical Data Science Lab
- Medical Deep Learning Lab
- Medical Data Engineering Lab
- Junior Research Group Diagnostics and Research of Movement Disorders